The Key To Better Business Decisions Isn’t Having More Data. It’s Having The Right Data.
A conversation with Insipher CEO David Cunningham on how organizations can improve how they use data to solve problems.
Lizzy Raben is a subscriber engagement editor at The Washington Post and is a Long Dash alum.
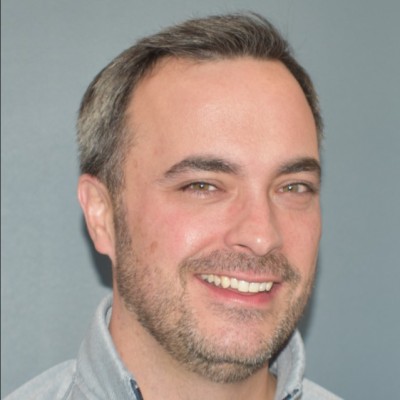
David Cunningham is the CEO and co-founder of Insipher, an open insights platform focused on empowering entire organizations to move from data chaos to comprehensive knowledge using machine learning. Prior to Insipher, Cunningham spent 20 years supporting advanced IT and major transformational programs within the Department of Defense, intelligence community, civilian agencies, and commercial clients. For transparency, Insipher is a Long Dash client.
Though organizations have more data than ever at their disposal, many still struggle to incorporate data into their daily decisions. And with the economic devastation brought on by the pandemic, business leaders are now being asked to make better, faster decisions with fewer resources.
For these leaders, data and machine learning can, and should, be an essential tool, argues David Cunningham, data architect and entrepreneur—but only if organizations have the right mindset. The key to making better decisions isn’t having more data, he says. It’s having the right data. In an interview with Long Dash, Cunningham shares how organizations can improve how they use data to solve business challenges, and how they can take a smarter approach.
This interview has been edited for length and clarity.
For business leaders looking to have a better understanding of what data can do for their organizations, where do you recommend they start?
Don’t start with the data. Start with the problem. Understand what that problem is, understand what you’re trying to solve for, and understand what it means to your organization. Start with that, and then look at whether you even have the right data to help you solve that problem.
One of the things that we talk to business leaders about a lot is that it’s not just about what’s happening inside of a single department or a single part of the organization—it’s about creating impact across the organization. For example, leaders should be asking themselves, “How does my IT department impact my HR department? How do these departments impact my finance department and overall quality of life for my employees?”
To identify the problem, business leaders need to look across the entire organization—every piece of it is a living organism that impacts the other piece. Once an organization starts to look at that, they start to understand how they can optimize one piece to optimize another and drive efficiencies into the whole organization.
A lot of companies recognize that data can be really powerful, especially when it comes to solving business challenges. Is it always better to have more data?
Absolutely not. We firmly believe that it’s about the right data for the right problem. An organization that’s trying to solve a specific problem needs to make sure they’re getting the data that solves that problem.
For one, this helps them from an overall cost and efficiency perspective because they don’t have data analysts or data scientists dealing with the data that could potentially be a red herring. As much as data sets are valuable assets, the people who are analyzing them are just as, if not more, valuable. It’s essential to make sure that their time and their experience is being leveraged to help to drive the right data into the organization.
The other piece is knowing where that data came from, whether it came from social media or my HR system or from some proprietary database that I’ve built. I want to know that an individual data scientist or data engineer has cleansed the data or transformed the data in some way to make it better. I want to know what systems have touched it because all of that feeds into the broader context of the makeup of the data. That not only gives me the right answers, but it also gives me some fidelity or trust in those answers.
We’ve spoken with you about how it can be hard to tell a story about why data is important and how to use it. How can organizations think about data more constructively?
For as much work as we do in terms of manipulating the data and analyzing the data, it means absolutely nothing if I can’t tell that story. We need right visualizations and the right way to walk a user through what we’re reporting so that they can make decisions.
Look at what’s happening in the world today. There are so many charts that people see on a daily basis around COVID-19 cases. And all of them are different. They’re at different points in time. They’re presenting things differently. If we’re not able to clearly tell a story and explain where the data came from and what algorithms were run to tell that story, how can I put any trust in that story?
So it’s not just about the data, it’s how we tell the story about the data. If we can’t tell that story, then how are organizations supposed to drive new decisions based upon that data into their processes or their workflows? And how do we know how well it worked?
Sign up for OnBrand
Our weekly digest featuring ideas on the future of brand.
Have the events of 2020 thus far altered or accelerated any trends in machine learning and artificial intelligence?
This year has shown what machine learning and artificial intelligence can do. Machine learning and AI capabilities had been developing mostly behind closed doors. But the pandemic has helped show the public, “Look, this is what is possible. Here is where we’re able to use machine learning and AI to be able to solve problems.”
When you look at the pandemic, the very first question that we always get is, “Can Insipher detect when the next outbreak of COVID-19 is going to happen?” Machine learning and AI can’t do that. But we can help with tracing. We can help with the data analysis from labs. But to get to a point where we can, without a doubt, predict, “The next case is going to happen here”—absolutely not.
There’s still a misunderstanding and a fear about AI because people have things like I, Robot and Terminator in their minds. But there’s a lot that AI can do to just help push people forward and solve problems faster so that you can focus on the harder problems that AI can’t solve, such as an ethical problem or dilemma. Really, AI is there to complement and support. It’s not there to take everything over.
Both COVID-19 and the protests following George Floyd’s murder have elevated conversations about systemic racism and the struggles of vulnerable communities in our country that are not always understood. Can you talk about how methods of data collection and algorithmic bias can contribute to this lack of understanding?
When people look at COVID-19 or systemic racism, they’re looking at it as a discrete problem. We need to look at these issues within a broader context and think about the problems that we’re trying to solve, and then think about how we start to bring data in.
Everything that we do has some level of bias in it, and all of those biases come into play when we talk about data collection and algorithms. One of the ways to get away from that is more transparency and explanation. There should be more people looking at what I’m doing and more cross-validation.
I want to be testing in broader spectrums of data sets and looking at ways to further refine and validate my algorithms on my machine learning models. I don’t want to be the one person that is bringing in the data, analyzing it, and giving you the results. If I do that, I am completely biased to the problem that I’m looking at and the answer that I believe I want to get.
The key to that is to have those checks and balances in place that enable other people to see what you’re doing. And, show your work. Tell me how you got to those results so that I can start to cross validate them and understand the story that you’re trying to tell.
Do you have any words of advice for business leaders looking to utilize data at their organizations?
First, be ready to stumble and potentially fail on your first start. And if you’re going to fail, fail fast. Be able to pivot quickly with different data sets or tools, understand what’s going on, and then tell that story.
Second, don’t hesitate to ask the hard questions. If you don’t know what someone is talking about in terms of a result or an algorithm, or how they got to a certain outcome, ask those hard questions. If someone’s not able to give you answers, have some skepticism and awareness that perhaps those results are not something that you can trust and base business decisions upon. Keeping the human in the loop will always be necessary, and at the end of the day you, as a leader, will be held responsible for decisions, not the computer.